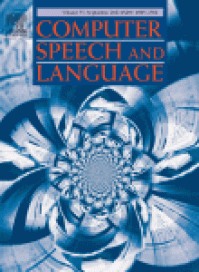
The article „Automatic screening of mild cognitive impairment and Alzheimer’s disease by means of posterior-thresholding hesitation representation" by José Vicente Egas-López, Réka Balogh, Nóra Imre, Ildikó Hoffmann, Martina Katalin Szabó, László Tóth, Magdolna Pákáski, János Kálmán and Gábor Gosztolya has been published in Computer Speech & Language.
Available online here: Egas-López, J. V., Balogh, R., Imre, N., Hoffmann, I., Szabó, M. K., Tóth, L., Pákáski, M., Kálmán, J. & Gosztolya, G. (2022). Automatic screening of mild cognitive impairment and Alzheimer’s disease by means of posterior-thresholding hesitation representation. Computer Speech & Language, 75, 101377. https://doi.org/10.1016/j.csl.2022.101377
Abstract:
Dementia is a chronic or progressive clinical syndrome, characterized by the deterioration of problem-solving skills, memory and language. In Mild Cognitive Impairment (MCI), which is often considered to be the prodromal stage of dementia, there is also a subtle deterioration of these cognitive functions; however, it does not affect the patients’ ability to carry out simple everyday activities. The timely identification of MCI could provide more effective therapeutic interventions to delay progression, and to postpone the possible conversion to dementia. Since language changes in MCI are present even before the manifestation of other distinctive cognitive symptoms, a non-invasive way of early automatic screening could be the use of speech analysis. Earlier, our research team developed a set of temporal speech parameters that mainly focus on the amount of silence and hesitation, and demonstrated its applicability for MCI detection. However, for the automatic extraction of these attributes, the execution of a full Automatic Speech Recognition (ASR) process is necessary. In this study we propose a simpler feature extraction approach, which still quantifies the amount of silence and hesitation in the speech of the subject, but does not require the application of a full ASR system. We experimentally demonstrate that this approach, operating directly on the frame-level output of a HMM/DNN hybrid acoustic model, is capable of extracting attributes as useful as the ASR-based temporal parameter extraction workflow was able to. That is, on our corpus consisting of 25 healthy controls, 25 MCI and 25 mild AD subjects, we achieve a (three-class) classification accuracy of 70.7%, an F-measure score of 89.6 and a mean AUC score of 0.804. We also show that this approach can be applied on simpler, context-independent acoustic states with only a slight degradation of MCI and mild Alzheimer’s detection performance. Lastly, we investigate the usefulness of the three speaker tasks which are present in our recording protocol.